Spatial PRIVACY in AUGMENTED REALITY
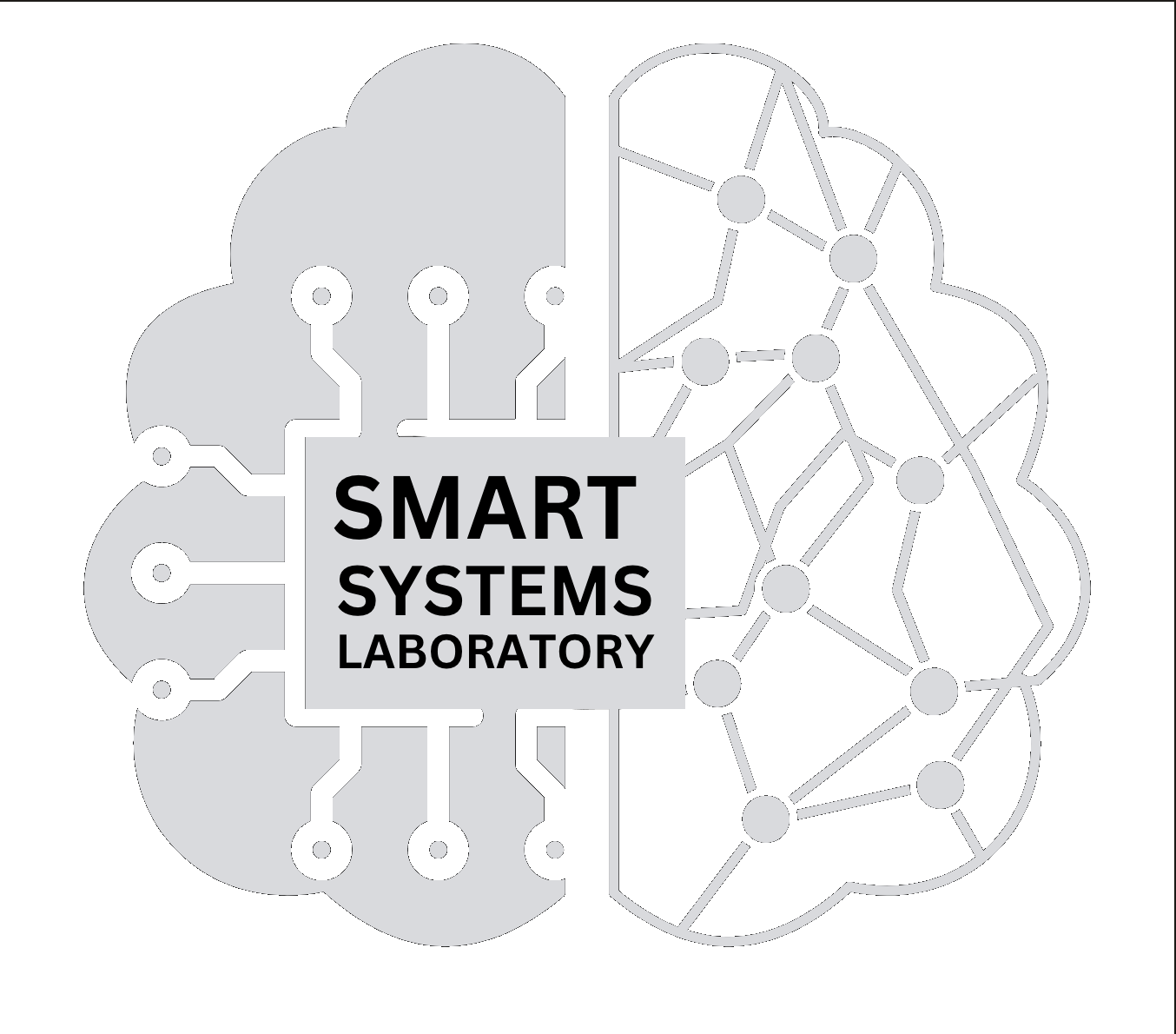
Jaybie A. de Guzman, Aruna Seneviratne, and Kanchana Thilakarathna, “Unravelling Spatial Privacy Risks of Mobile Mixed Reality Data”, Proc. ACM Interact. Mob. Wearable Ubiquitous Technol. 5, 1, Article 14 (March 2021).
https://doi.org/10.1145/3448103
Abstract. With the increasing user adoption of augmented, mixed, and virtual reality (AR/MR/VR; we collectively refer to as MR) technology on user mobile devices, spatial data has become more ubiquitous. However, this ubiquity also opens up a new threat vector for adversaries: aside from the traditional forms of mobile media such as images and video, spatial data poses additional and, potentially, latent risks to users of AR/MR/VR. Thus, in this work, we analyse MR spatial data using various spatial complexity metrics—including a cosine similarity-based, and a Euclidean distance-based metric—as heuristic or empirical measures that can signify the inference risk a captured space has.
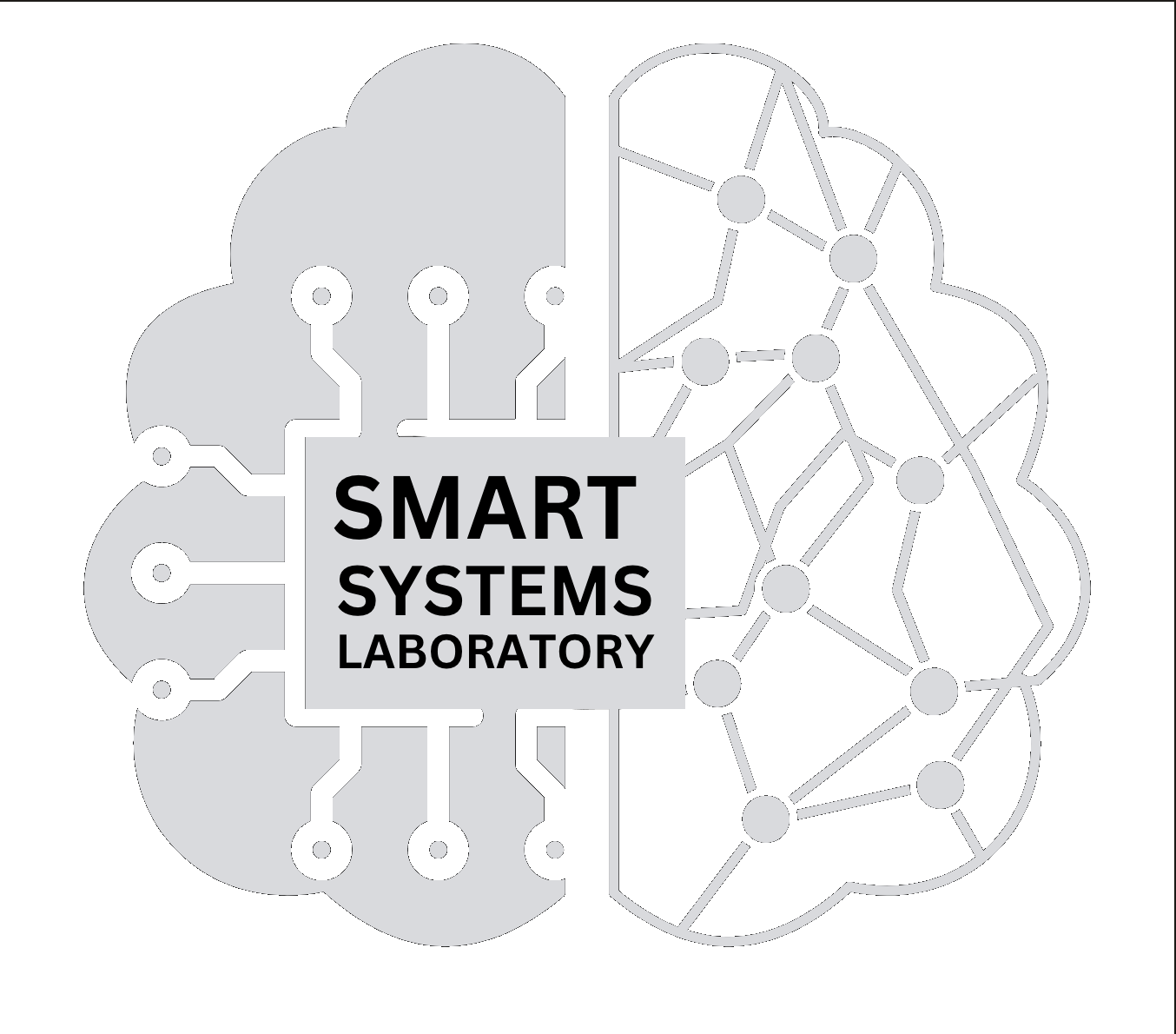
Arpit Nama, Amaya Dharmasiri, Kanchana Thilakarathna, Albert Zomaya, and Jaybie A. de Guzman. “User configurable 3D object regeneration for spatial privacy.” arXiv preprint arXiv:2108.08273 (2021).
Abstract. Environmental understanding capability of augmented (AR) and mixed reality (MR) devices are continuously improving through advances in sensing, computer vision, and machine learning. Various AR/MR applications demonstrate such capabilities i.e. scanning a space using a handheld or head mounted device and capturing a digital representation of the space that are accurate copies of the real space. However, these capabilities impose privacy risks to users: personally identifiable information can leak from captured 3D maps of the sensitive spaces and/or captured sensitive objects within the mapped space. Thus, in this work, we demonstrate how we can leverage 3D object regeneration for preserving privacy of 3D point clouds. That is, we employ an intermediary layer of protection to transform the 3D point cloud before providing it to the third-party applications. Specifically, we use an existing adversarial autoencoder to generate copies of 3D objects where the likeness of the copies from the original can be varied.